About Me
I am a Robotics Engineer specializing in autonomous quadcopters for educational applications at Duckietown.
I earned my BSc in Aerospace Engineering from the Polytechnic of Turin, where I developed my thesis under the supervision of Prof. Giuliana Mattiazzo. My work focused on designing telemetry systems for high-performance sailing boats used in competitive racing as part of the Polito Sailing Team.
I then pursued an MSc in Space Engineering at the Polytechnic of Milan, where I conducted my master’s thesis on reinforcement learning for planetary landing under the supervision of Prof. Michèle Lavagna during my internship at Deimos Space.
Additionally, I explored multi-agent SLAM and the adaptation of visuomotor policies during a research internship at the Toyota Technological Institute at Chicago, working under the guidance of Prof. Matthew Walter.
Research Interests
I am passionate about advancing robotics by integrating machine learning and control theory for real-world applications. My current interests include:
- Autonomous systems for aerial robotics.
- Reinforcement learning for adaptive control.
- Multi-agent SLAM and navigation in dynamic environments.
Publications
Davide Iafrate, Andrea Brandonisio, Robert Hinz, Michèle Lavagna,
Propulsive Landing of Launchers’ First Stages with Deep Reinforcement Learning,
Acta Astronautica, 2024,
https://doi.org/10.1016/j.actaastro.2024.11.028.
Projects
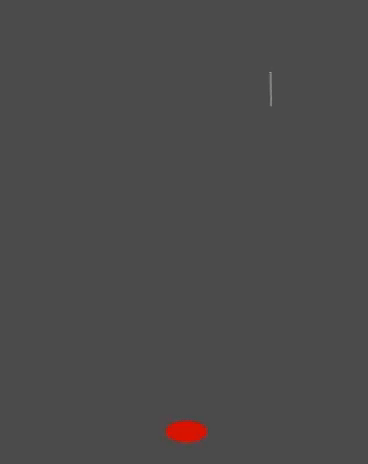
6DOF Planetary Landing Simulator
Reinforcement learning for 6DOF control in planetary landing simulations.
GitHub Repository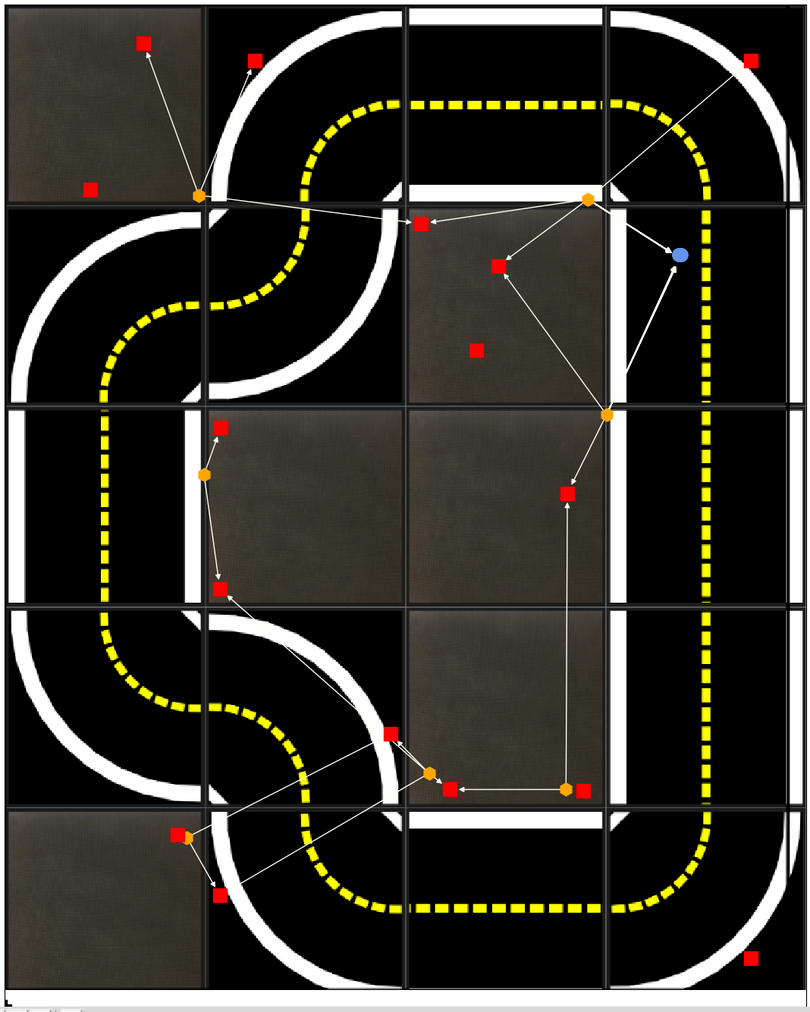
Multi-Agent SLAM
Research on collaborative SLAM for autonomous navigation in smart cities. I contributed to integrating odometry data from the vehicles to increase 3x the frequency of the localization estimate.
Website, GitHub Repository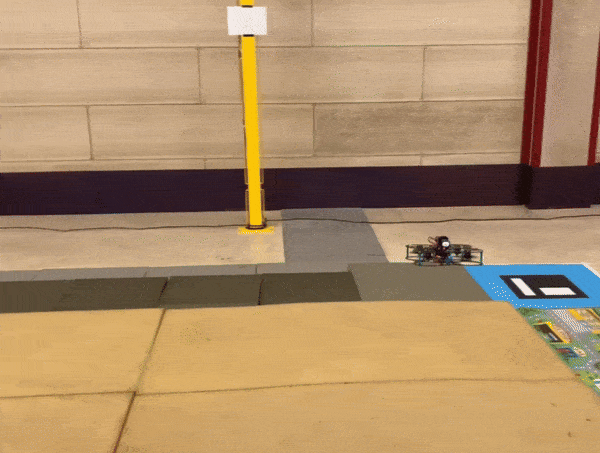
Adaptive MRAC Controller for Quadcopter
This project aimed to achieve trajectory tracking for a quadrotor using adaptive control laws by developing a baseline PI controller with LQR methodology, incorporating adaptive augmentation for handling uncertain aerodynamic effects, and introducing robust modifications to maintain performance under disturbances.
Report, VideoContact
- Email: davideiafrate@icloud.com
- GitHub: https://github.com/Tuxliri
- LinkedIn: Davide Iafrate